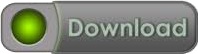

Numerous computer vision apps took advantage of residual neural network’s strong representational capabilities and noticed a massive boost. ResNet enables you to train hundreds, if not thousands of layers, while achieving fascinating performance. What Necessitated the Need for Residual Neural Networks?Īfter AlexNets celebrated a triumph at the 2012s LSVRC classification competition, deep residual network arguably became the most innovative and ingenious innovation in the deep learning and computer vision landscape history. A neural network that does not have residual parts has more freedom to explore the feature space, making it highly endangered to perturbations, causing it to exit the manifold, and making it essential for the extra training data recuperate. After this, the network eventually puts back the skilled layers while learning the feature space.Īs the training nears completion and each layer expands, they get near the manifold and learn things more quickly. Why? Because there are hardly any layers to spread through. It speeds up learning by tenfold, minimizing the effect of vanishing gradients. Skipping clears complications from the network, making it simpler, using very few layers during the initial training stage. It would be best if you considered using a Highwaynet in such cases. If that is not the case, utilizing a different weight matrix would be helpful for skipped connections. However, this only works effectively when all of the intermediate layers are linear or overlapping over the non-linear layer. In the most straightforward case, the weights used for connecting the adjacent layers come into play. While training, these weights adjust to the upstream layers and magnify the layer skipped previously. Most individuals do this by utilizing the activations from preceding layers until the adjoining one learns in particular weights. As the gradient is back-propagated to previous layers, this repeated process may make the gradient extremely small. The term used to describe this phenomenon is “Highwaynets.” Models consisting of multiple parallel skips are “Densenets.” Non-residual networks can also be referred to as plain networks when talking about residual neural networks.Ī massive reason for skipping layers is to steer clear of vanishing gradients and similar issues. Data scientists also take advantage of an extra weight matrix for learning the skip weights in some cases. The residual neural networks accomplish this by using shortcuts or “skip connections” to move over various layers.Įxperts implement traditional residual neural network models with two or three-layer skips containing batch normalization and nonlinearities in between. It assembles on constructs obtained from the cerebral cortex’s pyramid cells. A residual neural network referred to as “ResNet” is a renowned artificial neural network.
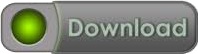